Continuous Learning in AI: Overcoming Catastrophic Forgetting
- muhammadzeeshan020
- Sep 1, 2024
- 3 min read
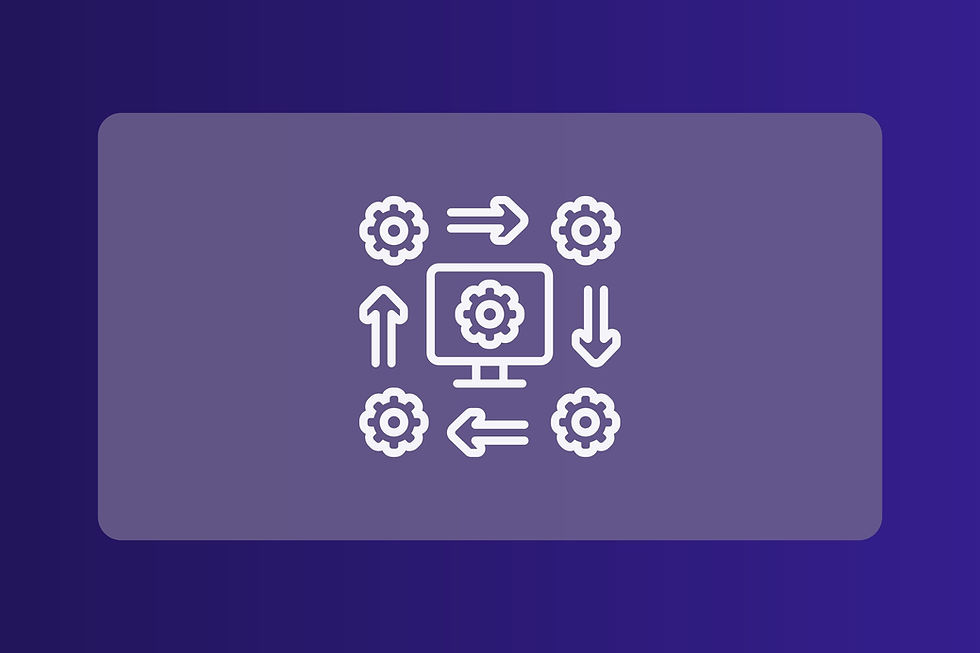
As artificial intelligence continues to advance, one of the key challenges in developing truly adaptive AI systems is enabling continuous learning while avoiding catastrophic forgetting. This blog post explores the concept of continuous learning, the problem of catastrophic forgetting, and various approaches researchers are taking to address this challenge.
Understanding Continuous Learning
Continuous learning, also known as lifelong learning or incremental learning, is the ability of an AI model to continuously update its knowledge and skills from a stream of data, without forgetting previously learned information. This capability is crucial for AI systems deployed in dynamic environments where new information constantly emerges.
The Challenge: Catastrophic Forgetting
Catastrophic forgetting occurs when a neural network abruptly and completely forgets previously learned information upon learning new information. This phenomenon is a major obstacle to achieving true continuous learning in AI systems.
Why it Happens:
Neural networks tend to overwrite old knowledge with new information
The distributed nature of neural network representations makes it difficult to update specific knowledge without affecting other parts
Approaches to Continuous Learning and Avoiding Catastrophic Forgetting
Researchers have developed various strategies to enable continuous learning while mitigating catastrophic forgetting. Here are some of the prominent approaches:
1. Regularization-based Methods
These methods add constraints to the learning process to preserve knowledge about previous tasks.
Elastic Weight Consolidation (EWC): Slows down learning on certain weights based on how important they are to previously learned tasks.
Synaptic Intelligence: Similar to EWC, but measures parameter importance during training instead of after.
2. Replay-based Methods
These approaches involve revisiting previous experiences to maintain performance on older tasks.
Generative Replay: Uses a generative model to produce pseudo-samples of previous tasks.
Experience Replay: Stores and replays real samples from previous tasks during new learning.
3. Architectural Strategies
These methods involve modifying the neural network architecture to accommodate new knowledge without interfering with old knowledge.
Progressive Neural Networks: Adds new neural "columns" for new tasks while preserving old ones.
Dynamic Expandable Networks: Dynamically expands the network capacity when new information cannot be incorporated into the existing structure.
4. Meta-Learning Approaches
These strategies aim to learn how to learn, making the model inherently more adaptable to new tasks.
Model-Agnostic Meta-Learning (MAML): Trains the model to be easily fine-tuned to new tasks with minimal data.
Learning to Learn: Develops internal representations that are broadly applicable and easily adaptable.
Recent Research and Advancements
Continual-BERT: Researchers have adapted the BERT language model for continual learning, allowing it to learn new tasks without forgetting old ones.
Gradient Episodic Memory (GEM): This method uses an episodic memory to store past experiences and constrains gradient updates to avoid interfering with past tasks.
Conceptual Expansion: This approach dynamically expands the conceptual space of the model to accommodate new knowledge while preserving existing concepts.
Neuromodulated Plastic Networks: Inspired by biological brains, these networks use neuromodulatory signals to regulate synaptic plasticity, allowing for more flexible learning.
Challenges and Future Directions
Despite significant progress, continuous learning in AI remains a complex challenge. Some areas of ongoing research include:
Developing more efficient memory mechanisms for storing and accessing past experiences
Creating better metrics for measuring catastrophic forgetting and continuous learning performance
Exploring the balance between stability (preserving old knowledge) and plasticity (acquiring new knowledge)
Investigating how to transfer knowledge between tasks more effectively
Addressing the scalability of continuous learning approaches to large-scale, real-world applications
Conclusion
Continuous learning is a critical capability for creating truly adaptive AI systems. By addressing the challenge of catastrophic forgetting through various innovative approaches, researchers are paving the way for AI models that can learn and adapt throughout their lifetimes, much like biological intelligences. As this field continues to evolve, we can expect to see AI systems that are increasingly capable of learning and adapting in dynamic, real-world environments.